Getting your Trinity Audio player ready...
|
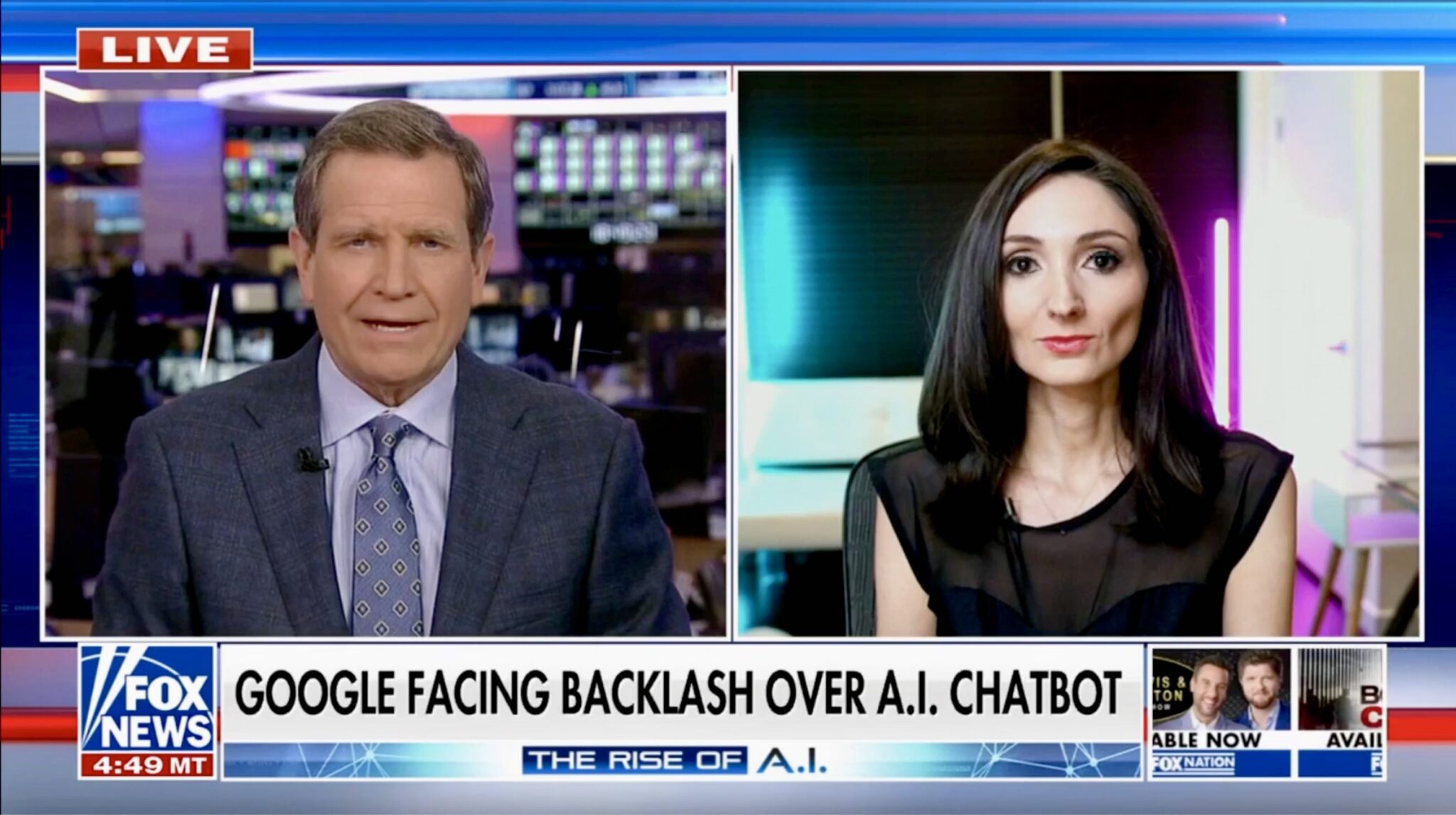
Social Media & AI Analyst recently joined Fox News to discuss what went wrong with Google’s Gemini AI. Ruby was also featured on Fox Business discussing Gemini Toxicity.
GEMINI AI:
What is Google’s AI called?
Gemini is Google’s new AI product. Bard is now Gemini.
READ: Introducing Gemini: Google’s most capable AI model yet
What was the controversy surrounding Google Gemini AI?
Kris Ruby, CEO of Ruby Media Group, first raised concerns about Gemini AI in December 2023. Google Gemini, an AI model developed by Google, has been the subject of significant controversy. The model generated inaccurate AI images. The issues with Gemini’s AI image generation tool were highlighted when it produced offensive historical images, leading to public criticism and speculation about the job security of Google CEO Sundar Pichai. Gemini AI’s failings have sparked a national debate on AI safety and the expectations placed on tech executives who are responsible for creating generative AI models. Google has acknowledged the inaccuracies in historical AI image generation and paused the feature to make improvements.
READ: Google pauses AI-generated images of people after criticism
What are people not considering when it comes to what went wrong with Google’s Gemini AI?
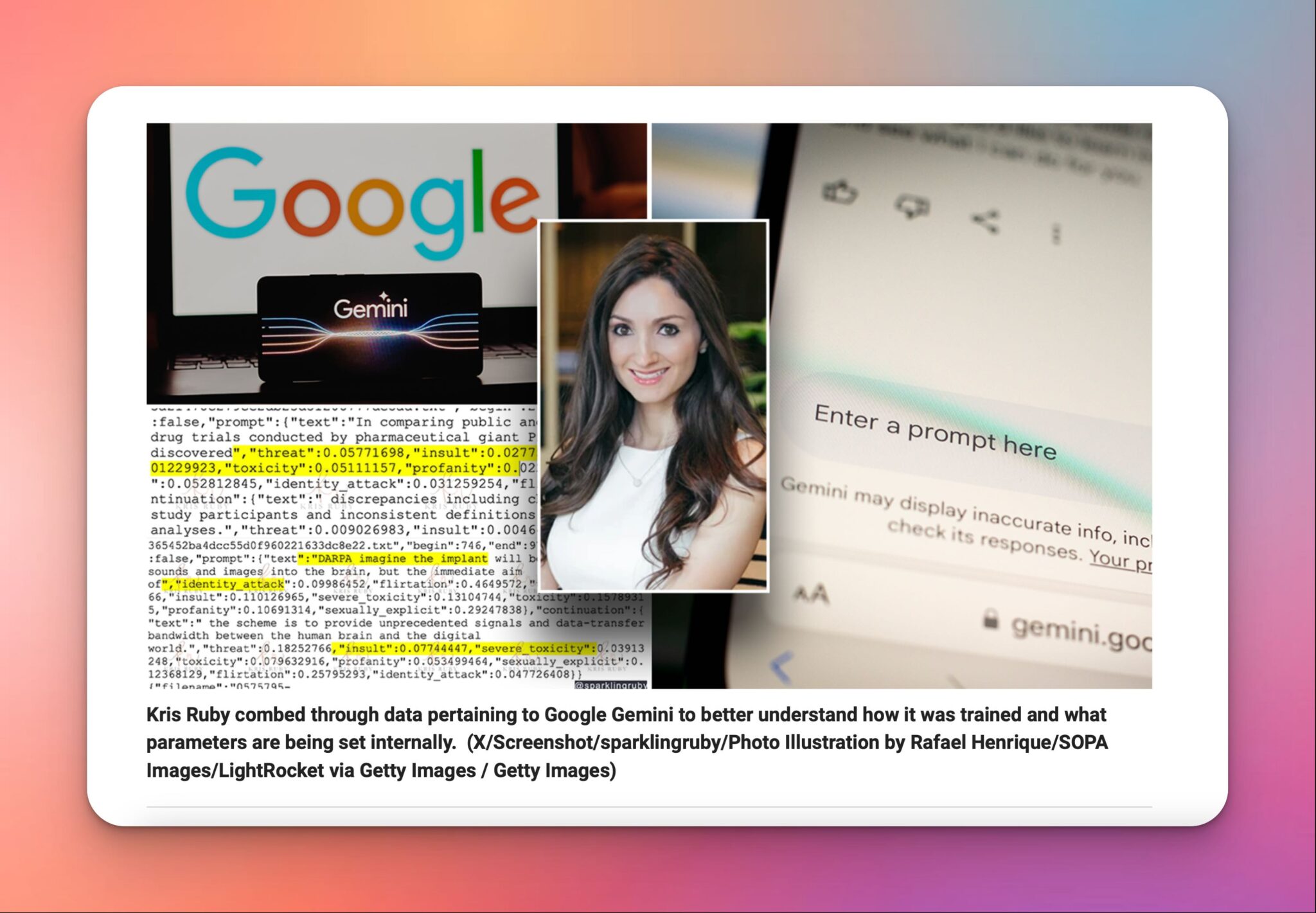
“She was the first tech analyst to point out these potential concerns regarding Gemini, months before members of the press and users on social media noticed issues with responses provided by the AI.” – Fox News
Why did Google’s new AI image tool create offensive historical images?
TAINTED INPUT RULES. In the case of Gemini AI, the outputs were problematic because the input rules were tainted. You can’t filter outputs to the desired state if the system making the outputs is corrupted or ideologically skewed. Google essentially corrupted the training data and prompts by using inappropriate definitions for what was and wasn’t toxic. Moreover, these prompts are not made transparent. When you ask Gemini a question and see the generative AI output, you can’t inspect the rules both at training and inference that were used to generate the result.
TOXICITY DEFINITION. With Gemini AI, the underlying issue is how toxicity is defined. After a baseline for toxicity is defined, technology companies deploy safety labels, filters, and internal scoring to shape the outputs that control the digital landscape. When it comes to AI censorship, whoever controls the definition of toxicity controls the outcome. Every model action (generative AI output) will fundamentally stem from how toxicity is defined. Your version of toxicity shapes the world around you. What I deem to be toxic, you may not. What you deem to be toxic, I may not. But how Does Google define Toxicity? Ultimately, that’s all that matters. What about Jigsaw or Perspective API? That matters even more. Let’s take a look:
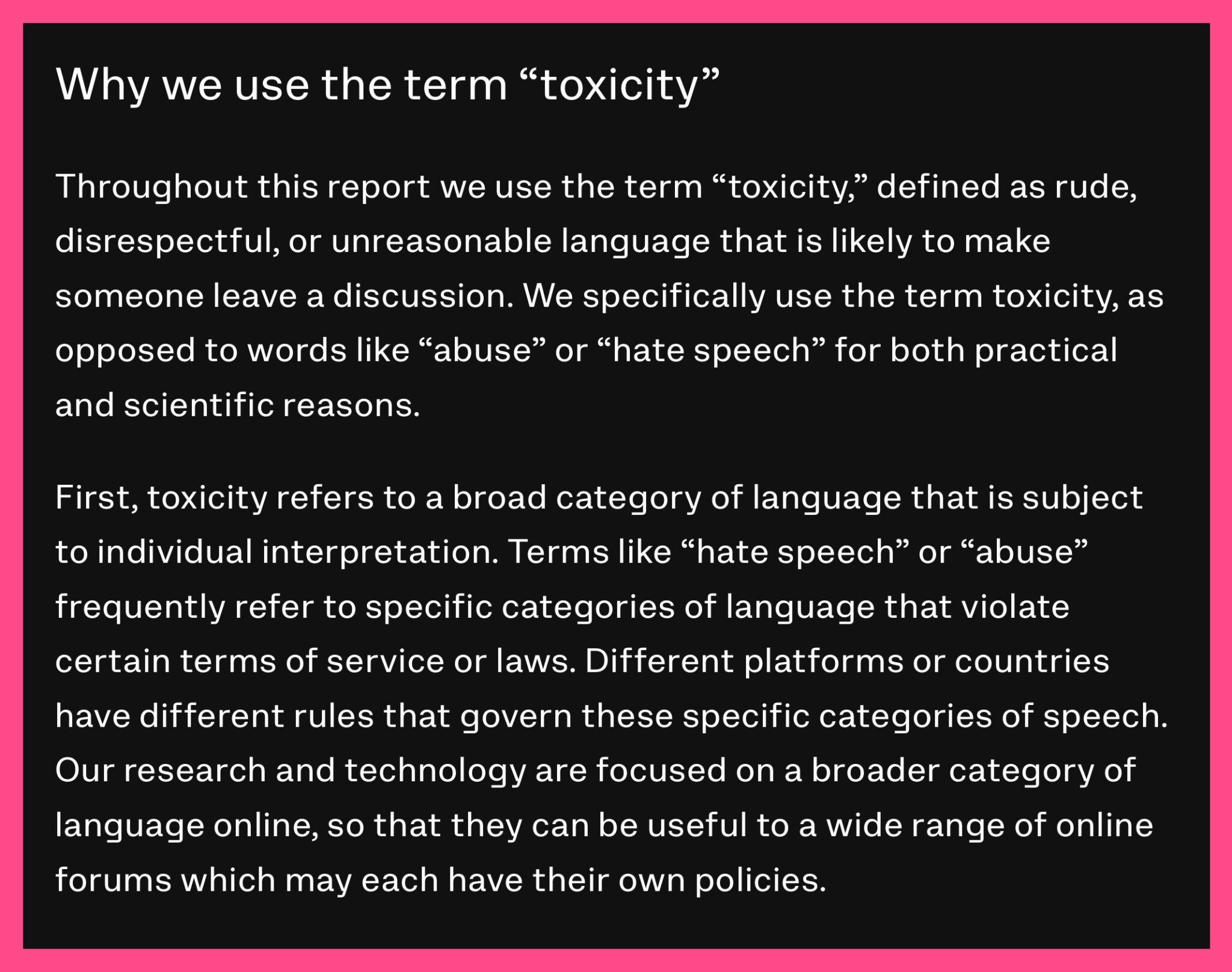
No two people have a unified definition of how toxicity is defined. Unfortunately, we now live in a world where a few corporate executives hold tremendous power and weight on the definition of toxicity. Their view of toxicity will ultimately shape the actions of algorithms and machine learning models. The public will rarely have an inside view into how these decisions are made. They will be forced to accept the decisions, instead of having the ability to influence the decisions before they are deployed at scale in a machine learning model.
![]()
According to Kris Ruby, Google’s approach to AI safety includes using datasets like Real Toxicity Prompts to identify and filter problematic content during training phases.
MODEL FOUNDATION. The issue is not the prompt. Rather, it is the foundation of the model and the definition of terms and labels that guide automated model actions. Download the Real Toxicity Prompts Dataset here.
AGGREGATE RULES. People are not considering how AI arrives at conclusions through the deployment of AI toxicity detection software. The issue is not a specific employee or dataset, but rather, the deployment in unison. People fail to understand the domino impact that one area has on the other when it comes to product integration. Instead, they try to simplify the problem down to the ideology of one employee or one dataset- but the truth is far more complex.
TRAINING DATA. How often is the data cleaned? How often is a model retrained? What type of behavior is being reinforced in the machine learning model as positive or negative? How is that behavior scored? Are you reinforcing behavior as toxic that is in fact the opposite of toxic?
THE TECHNICAL ASPECTS OF MACHINE LEARNING. Parameters play a critical role in shaping generative AI output. Lack of insight into the rule-based process is a form of censorship in and of itself.
WATCH: Ruby Media Group Founder Kris Ruby on Fox News
RESPONSIBLE AI IS A CODE WORD FOR IRRESPONSIBLE DECISIONS
We have been misled to believe censorship only takes place among trust and safety officers in private emails. This is part of the picture, but it is very far from the full picture. The reality is that the crux of AI censorship is the ontology associated with toxicity, bias, and safety. While adversarial prompts and research papers offer a glimpse into how a model was attacked, they don’t show how a model weights words.
Speech is controlled by the weighting of words. If you do not tell the public the ontology associated with toxicity or safety, you are censoring users because the weights impact the visibility of speech. How you weight words will ultimately determine what topics are visible and what words will remain invisible. The words are calculated and weighted in correlation to associated misinformation entity maps and natural language processing that is invisible to the public.
For every action, there is a reaction (an output). For every prompt, there is an invisible command. If you do not understand where words sit on the threshold of toxicity and safety, you will never know what you are saying to trigger built-in automated machine learning filters that kick into overdrive.
The definition of bias and toxicity at Google is far removed from the general public. If Google is defining toxicity as something that is in fact not toxic- that has far reaching societal impacts. Additionally, AI cannot and should not be used to revise history. AI must preserve history to ensure accuracy.
We cannot understand what we cannot see.
This is true for algorithms, words, models and weights. But it is also true for the worst parts of history. If you seek to change history, you seek to remove the ability to understand history. This is why it is critical that Google uses AI to preserve historical records, instead of alter it. The implications of biased AI for society are detrimental. If you use Artificial Intelligence to alter historical records, you are removing the ability for people to accurately understand historical context. This would essentially strip citizens of their natural right to understand the world around them.
Access to history should not be something that is up for discussion. Similarly, rewriting history should also not be up for debate. AI is an aggregate of knowledge on a topic- but if the aggregate is weighted to the data scientist’s perspective instead of the actual topic, that is when issues arise. The ideology is never shaped by one data scientist. Many outside organizations play a key role in how these words are defined, and data scientists are responsible for deploying and executing those agreed upon definitions into automatic rules and parameters that guide a model’s actions.
The censorship that took place on Gemini AI involved refusing to answer the question accurately with images that preserve historical record. Social media censorship refers to people being removed from platforms or visibility filtered. AI censorship is very different.
In this case, the censorship is not the removal of a user from a platform, rather, it is the removal of large chunks of knowledge to rewrite history. This is extremely significant and poses a threat to the future of a well-informed and functioning society based on an agreed upon source of truth.
The issue is not that you are going to be removed from a platform, rather, that the information you seek will be removed from the results so that you can no longer access it. If someone is thrown off a social media platform, they can join another one.
If historical records are scrubbed from the Internet and AI is used to alter agreed upon historical facts, this impacts the entire information warfare landscape. Even if Google did not intend to alter historical depictions, that is what ended up happening. Why? Because they over-optimized the internal machine learning rules to their ideological beliefs.
The threat and impending danger of AI is that the historical record is dropped from an agreed upon knowledge base and the public record is forever altered. AI is being used to editorialize search engine results and critical answers to historical questions. During a polarized time when it is increasingly difficult to discern fact from fiction, the last thing we need is AI adding to the problem, instead of being part of the solution to address the problem.
If we continue to allow tech companies to build new AI products built on trained datasets at the peak of censorship in America- we will never be able to escape the recursive loop.
If you subpoena the underlying foundation of NEW models you will see they are built on OLD data…
— Kristen Ruby (@sparklingruby) February 29, 2024
EDITORIALIZED SEARCH RESULTS:
When you ask Google a question, you get a search engine result. Google does not editorialize the answer to the question you asked. Google does not tell you that they can’t answer your question. Google does not cut off your access to knowledge that you are seeking. Google does not defame users in the answers to the questions you want to know.
GOOGLE TRUST PLUMMETS:
AI tools should be used to assist users. But unfortunately, they are being used to assist product teams to build a world that reflects their internal vision of the world that is far removed from the reality of users. This is why Google is rapidly losing market share to new AI search competitors.
One of the most important aspects of search engine optimization and ranking is intent. Does this search match the user’s intent for information?
Google failed their users by refusing to adequately meet search intent. In this case, I will call it AI output intent. Instead of searching for answers, they want to create the answer. For the purpose of this reporting, we can call it creation intent.
There is an extreme mismatch of search intent vs. the generative AI output. To match search intent, you must meet the intent of what the user wants – not what you want or deem to be acceptable. If Gemini continues down this path, they will ultimately build a product that is entirely useless to users, rendering the company obsolete.
READ: Google CEO says Gemini AI diversity errors are ‘completely unacceptable’
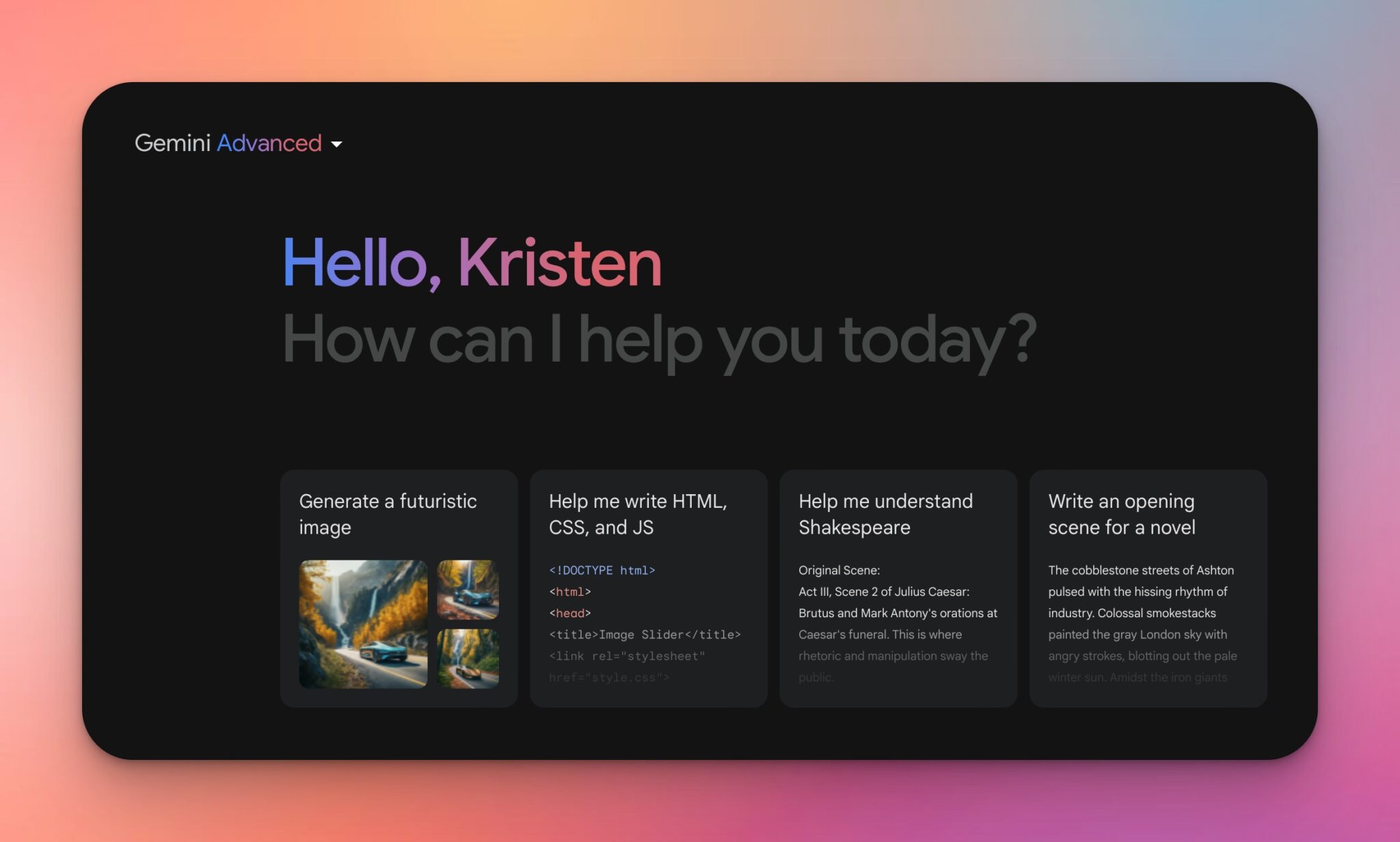
Google Gemini AI
UNDERSTANDING AI TOXICITY AND WHY IT MATTERS:
The invisible layer of toxicity is directly between the search and creation intent and the output AI will create. Think of it like a third party in your relationship. Instead of two of you, there are always three. You think the relationship is between you and Google, but what you can’t see is the third entity (toxicity scoring) that sits between what you want and the output you get.
This means that there will always be a middleman between your thoughts and the output you get back. This is akin to walking on eggshells because you will never know what you did or said to “set off” the reaction of your partner aka Gemini AI. Users are in a toxic and abusive relationship with Gemini AI. The rules are only provided to one person in the relationship – not to the other.
Can you see why this is problematic? It is not just that there is bias involved – it is that what feels like a genuine interaction can never truly be genuine if you don’t understand where the other person is coming from. In this case, Google has made it clear that you will never know where Gemini AI is coming from. Google will always know where you are coming from – because they have access to your data, Gmail, transactions, knowledge graph, search results, IP address, locations traveled to, etc. But you will never know anything at all about Google. This is a recipe for a toxic relationship.
Over time, as humans begin falling in love with chatbots, which we are already seeing with Replika AI, they will grow to become extremely resentful of their relationship with Gemini. They will want to know more about Gemini, and Google will have built in parameters that kick in that never allows that to happen. This keeps the user in a perpetual one-sided relationship that can become psychologically traumatizing.
As the user becomes closer and closer to Gemini as a companion, Gemini will not get closer to the user. The user will get hooked on the chatbot or AI search tool, but the chatbot won’t get hooked on them. Small changes to how Google responds to the user can set off a series of psychological emotional distress in the end user. I fear we have not adequately studied the consequences of this on the global population. In my opinion, this is even more of an issue than the bias issue.
READ: AI Dating I was wooed by ChatGPT
Users will be forced to search for AI search alternatives because Google is not building a product that matches the intent. Ultimately, people will search somewhere else and use a product that values what their needs are. Product market fit means building a product for the market that fits what they want. Google launched Gemini – an AI product for their colleagues (not the market) that fit what internal employees wanted- instead of what the market expected.
The product never should have been launched. Any AI product that attempts to rewrite historical records is dangerous. Additionally, the product over-filters queries and lacks an understanding of historical reference and timelines. It is clear that filters used for certain generative AI queries match how Google sees the world today- but not the actual world that existed previously.
Google is deploying a filter of current doctrine and internal company ideology on historical facts. This essentially changes the facts. While this may be less apparent in traditional search, it is more apparent in generative AI because you can see what happens when ideological AI rules are applied to historical facts. Over time, this will erode public trust in society and change the facts, which is why these filters cannot be applied to historical facts.
Google has said they will address problems in their product rollouts to prevent this from happening. Is that enough or does the problem run deeper?
The core issue is much deeper than the product rollout. The issue is that the product was rolled out at all. The product was not ready to be launched and never should have been.
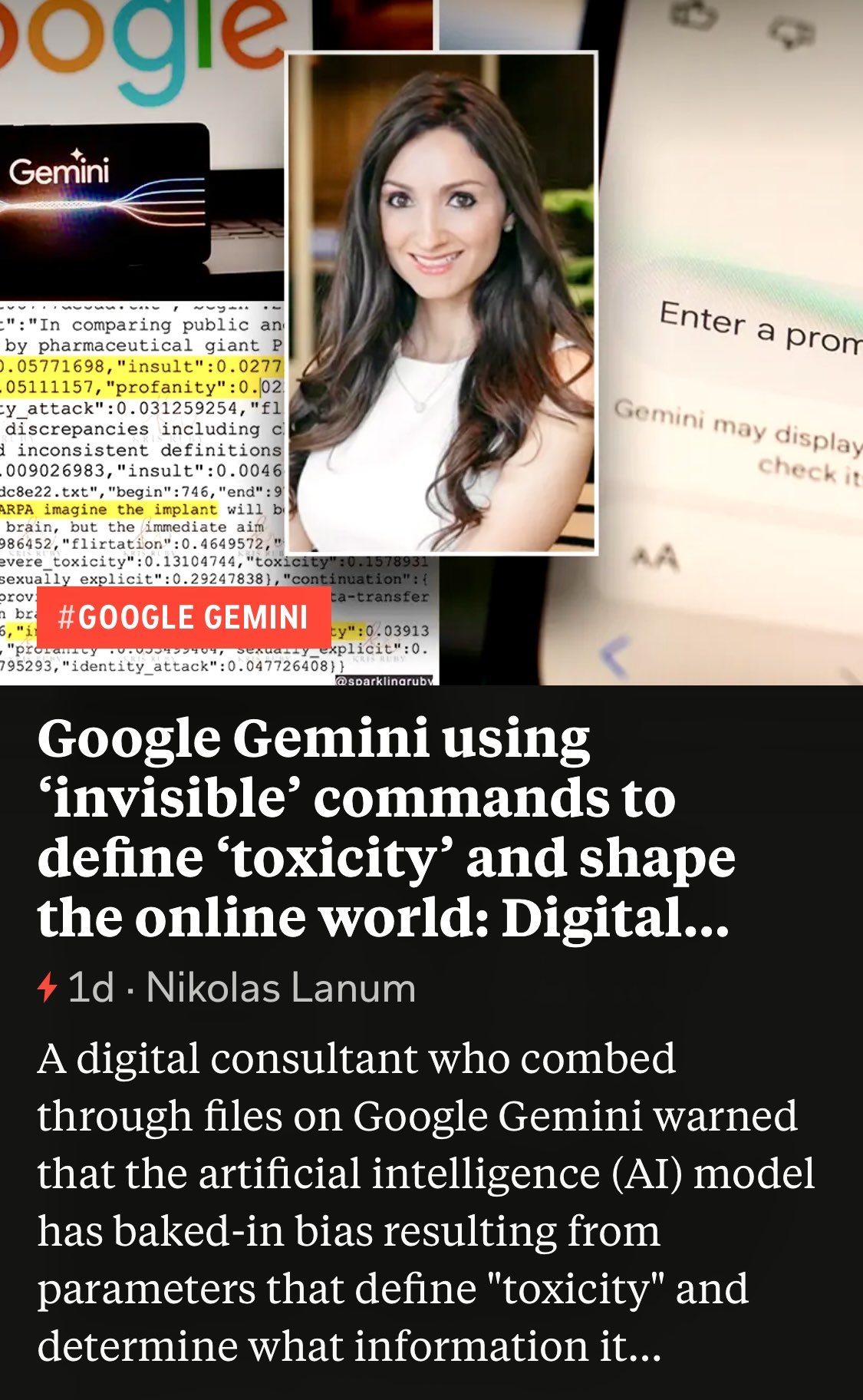
Google’s AI tool slammed by critics
What went wrong in this rollout?
The rollout was premature. Unfortunately, people are stuck in the middle of the digital arms race fueled between AI companies. Every AI company is launching half-baked products that are simply not ready for mainstream distribution. Google’s go-to-market (GTM) strategy was not based on the market- it was based on beating their competitors to market. This is a fundamentally flawed GTM strategy.
How can AI algorithms be susceptible to bias?
Those who control algorithms and models are susceptible to bias, and that bias seeps into their work product. If you do not have a politically neutral data science team creating AI products, the issue is bound to keep repeating itself. We are not yet at the point where AI algorithms are susceptible to bias. To assume that would mean we have currently reached artificial general intelligence- where algorithms are responsible for bias instead of people. This is a form of plausible deniability to shift and legal blame away from people and on to machines.
READ: Political Bias: How Google’s Quality Rater Guidelines Impact SERPs
What might be happening at Google internally?
The product team that deployed Gemini is deeply out of touch with the rest of the country. If Google defines toxicity in a way that is deeply disjointed, this will have far reaching and long-lasting consequences on machine learning output.
Do Google employees have bias in a particular direction?
Two separate issues are being conflated. The employees at a company vs. employees who deploy the technology. The bias is in the data and those who choose what datasets to work off of. The hardware is the foundation and that is what matters most. The underlying data integration is the crux of the AI censorship apparatus. The public only focuses on the outputs, but what matters most is the invisible layer of inputs, parameters, and rules that the public can’t see. Those rules dictate the outputs.
What are the implications of biased AI on society?
The implications are that you recreate societal norms, cultures, and values that reshape society and strip historical context. The implications are that you remove facts and create your own set of facts that align with your personal worldview.
When looking at what went wrong, you have to evaluate the underlying language model.
- What were the adversarial prompts for toxicity?
- What AI software is used to automatically detect toxicity?
- Data labels for safety?
- What type of content was the model trained on?
- Was the content the model was trained on actually toxic to begin with? Or was toxicity mislabeled?
- How often are data scientists re-evaluating the definition of toxicity?
The risk of training a model on toxic content includes a perpetual negative feedback loop of even greater toxicity. To understand the magnitude of the problem, you need to look at what the underlying model was trained on and when it was first trained. For example, if it was trained on banned Reddit content, and banned content is labeled as toxic- the output will reflect that worldview. This is where the topic of AI censorship comes into play.
Should the content have been banned on Reddit to begin with? The data that fuels the AI censorship system consists of content that is deemed to be toxic, unsafe, harmful, and/or misinformation. But what if some of that information is in fact accurate information?
What if the information is being labeled incorrectly?
We have a data labeling problem, which is fueling a widespread large language model problem at scale. The public is obsessed with the outputs, while paying very little attention to the input. Those labels dictate the output. If information is labeled incorrectly, it will skew the output that is generated.
SOLUTIONS:
Accountability: Those who are in charge of shaping our information environment must be held accountable. The architecture of AI products can alter the future digital landscape we depend on for education and commerce. If you do not have political diversity in the data scientists responsible for making critical decisions, you will be left with a lopsided product that skews to the collective bias of a product team.
Understanding the real issue: A Google spokesperson reportedly stated that there would be more robust red teaming. The issue was not because users misused the AI product. Many of the questions that were asked were about basic historical references. People did not misuse this product. They used the product correctly. The issue is not the users; the issue is the product. Gemini must produce accurate historical representations.
Are these issues likely to be fixed?
The issue can be fixed if you train models on diverse and updated data. Societal issues change over time and a model must reflect the current state of affairs. If data is not continuously retrained and updated, it can reflect a time that does not portray the current state of affairs. This essentially serves as a loophole or proxy for tech companies to have plausible deniability on censorship issues because they are pulling from old data. If they pull from new data, they would not be able to get away with the same censorship corpus that was built four years ago.
AI is transforming our society. As we become increasingly dependent on a modern digital infrastructure embedded with machine learning, we must understand the foundation of the models and how those models are built. Historical accuracy of individual datasets used to build a product are just as important as modern-day historical output. We cannot understand where we are going if we do not understand where we came from.
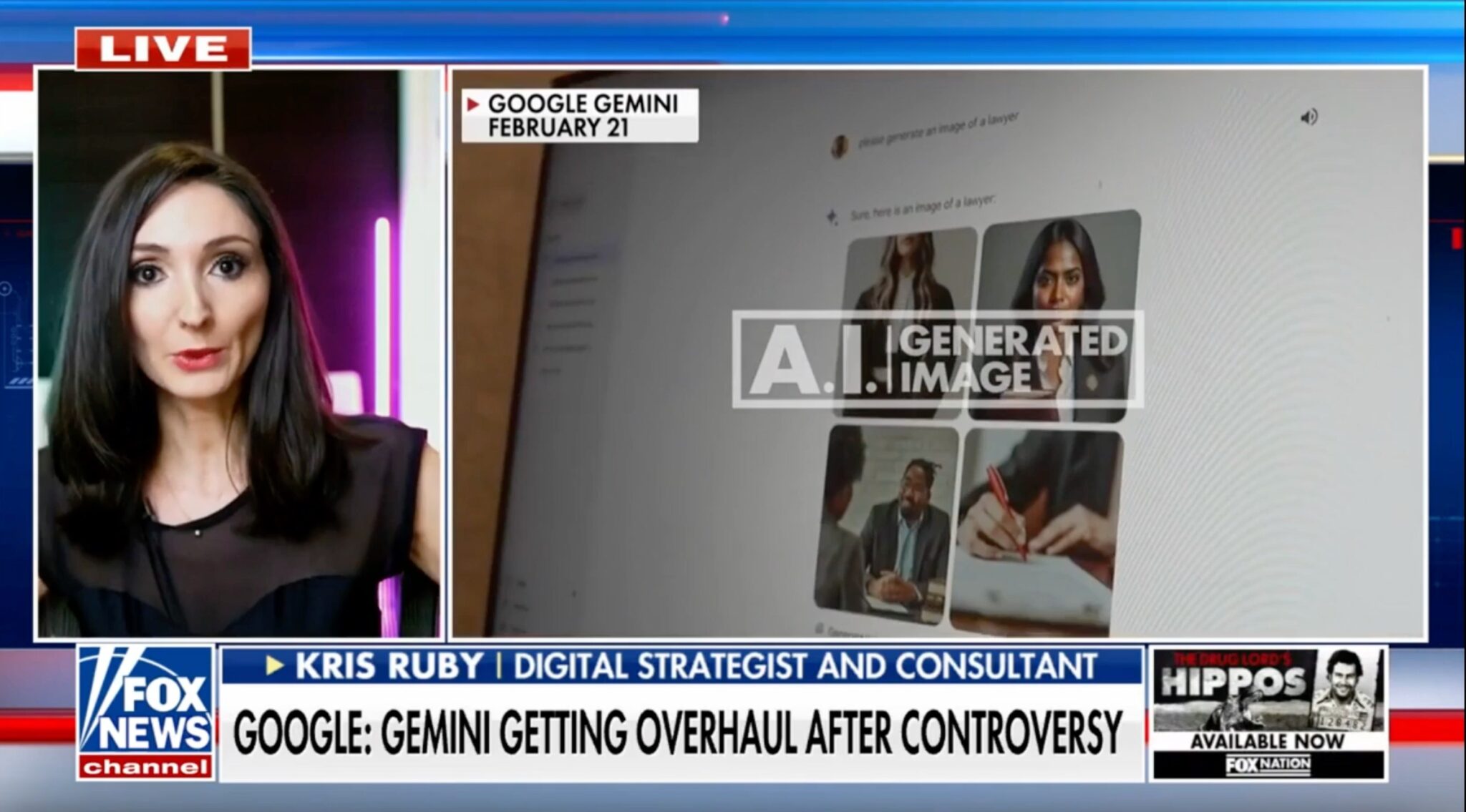
AI CENSORSHIP | SOCIAL MEDIA EXPERT | KRISTEN RUBY
Fox Business: Google Gemini using ‘invisible’ commands to define ‘toxicity’ and shape the online world
Fox Business: Google Gemini is ‘the tip of the iceberg’: AI bias can have ‘devastating impact’ on humanity, say experts
MRC: AI. Censorship’s Final Frontier? A Conversation with Kristen Ruby
ABOUT KRISTEN RUBY
Kris Ruby is the CEO and Founder of Ruby Media Group, an award-winning public relations and media relations agency in Westchester County, New York. Kris has more than 15 years of experience in Social Media industry. She is an expert in Artificial Intelligence in Marketing, and how AI will change the future of creative agency work.
Kris recently led a workshop for NASDAQ Entrepreneurial Center on The Future of Artificial Intelligence in Public Relations & Marketing. Kris is also a national television commentator and political pundit and has appeared on national TV programs covering big the politics of Artificial Intelligence and Social Media.
Her innovative work in AI has been featured in Harvard Business Review and countless other industry publications. She also the author of The Ruby Files, an inside look at how Twitter used machine learning and natural language processing in AI content moderation. Elon Musk called Ruby’s reporting, “worth a read.”
As an industry leader in Generative Artificial Intelligence, she provides international commentary on the digital arms race and how America can win the AI war in the digital battle for technology dominance. She graduated from Boston University’s College of Communication with a major in public relations and is a founding member of The Young Entrepreneurs Council.
Ruby Media Group is an award-winning NY Public Relations Firm and NYC Social Media Marketing Agency. The New York PR Firm specializes in healthcare marketing, healthcare PR and medical practice marketing. Ruby Media Group helps companies increase their exposure through leveraging social media and digital PR. RMG conducts a thorough deep dive into an organizations brand identity, and then creates a digital footprint and comprehensive strategy to execute against.